The giant robot in the corner would like a word, or 500. It’s been standing there quietly for a while, helping out with mundane tasks — sorting and filing, looking up info and the like — generally staying out of the way unless called on. You might not have even noticed it. But you will.
Earlier this year, the text-to-image generation tools Stable Diffusion, Midjourney, and DALL-E 2 made impressive public debuts. You’ve no doubt seen the results in your social media feeds. And just this month, OpenAI released ChatGPT, a chatbot based on its unsettlingly smart GPT-3 large language model — quickly driving home both the promise and peril of generative AI.
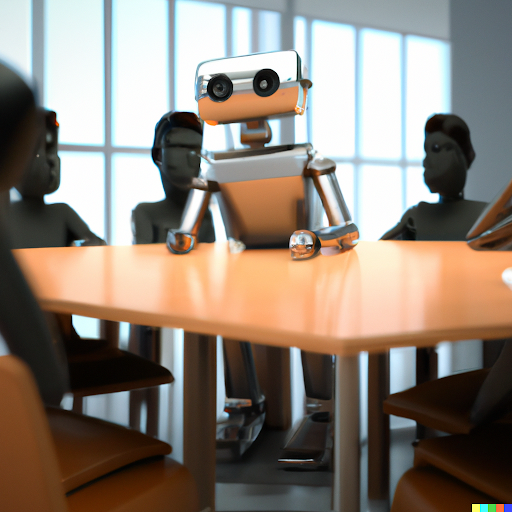
Made with DALL-E 2: “Illustration of a robot sitting awkwardly at a conference table in a newsroom alongside several editors, who look concerned.” (A human illustrator would no doubt have done this better.)
It’s hard to overstate the disruptive potential of the machine-creative revolution we are witnessing — though some are clearly trying: “The death of artistry.” “The end of high-school English” (and the college essay, too, evidently). We now have non-developers producing functional code and a children’s book written and illustrated entirely by machine.
Of course, AI tools have been all over our industry for a while now: We’ve used them for transcription, translation, grammar checking, content classification, named entity extraction, image recognition and auto-cropping, content personalization and revenue optimization — among other specific purposes.
But emerging use cases made possible by generative tools — including text and image creation and text summarization — will broaden the scope of AI’s impact on our work.
I don’t imagine we’ll see GPT-3-produced copy in the pages of The New York Times in 2023, but it’s likely we’ll turn to machines for some previously unthinkable creative tasks. As we do, we will hopefully reflect on the risks.
Even the best generative AI tools are only as good as their training, and they are trained with data from today’s messy, inequitable, factually challenged world, so bias and inaccuracy are inevitable. Because their models are black boxes, it is impossible to know how much bad information finds its way into any of them.
But consider this: More than 80% of the weighted total of training data for GPT-3 comes from pages on the open web — including, for example, crawls of outbound links from Reddit posts — where problematic content abounds.
Add to that the tools’ disconcerting habit of obscuring sources and presenting wildly incorrect information with the same cheery confidence it applies to accurate answers, and you have high potential for misinformation (to say nothing of the dangers of deliberate misuse).
ChatGPT is incredibly limited, but good enough at some things to create a misleading impression of greatness.
it’s a mistake to be relying on it for anything important right now. it’s a preview of progress; we have lots of work to do on robustness and truthfulness.
— Sam Altman (@sama) December 11, 2022
Will these tools get better? Undoubtedly. We may be in something of an uncanny valley stage, and who knows how long that will last?
Right now, though, my take is this: For all its promise, generative AI can get more wrong, faster — and with greater apparent certitude and less transparency — than any innovation in recent memory. It will be tempting to deploy these tools liberally, and we know that some black-hat SEOs will be unable to pass up the opportunity to publish thousands of seemingly high-quality articles with zero human oversight. But the possible uses will always be more numerous than the advisable ones.
(And what will happen to model training, fact-checking, and general user experience when more and more of the information on the open web is produced by AIs? Will the web become one big AI echo chamber? OpenAI is already trying to build watermarking into GPT-3 to facilitate the detection of AI-generated text, but some experts believe this is a losing battle.)
Applying these powerful tools surgically to narrowly-defined use cases, while keeping humans in the loop and providing needed sourcing transparency (and credit!), will enable us to wield them for good.
But if we fail to build the necessary checks on AI’s creations, then the likelihood of students passing off robot-written text as their own will be the least of our worries.
Eric Ulken is a product director at Gannett.